Enhancing the Private Equity Business Model with Data Analytics
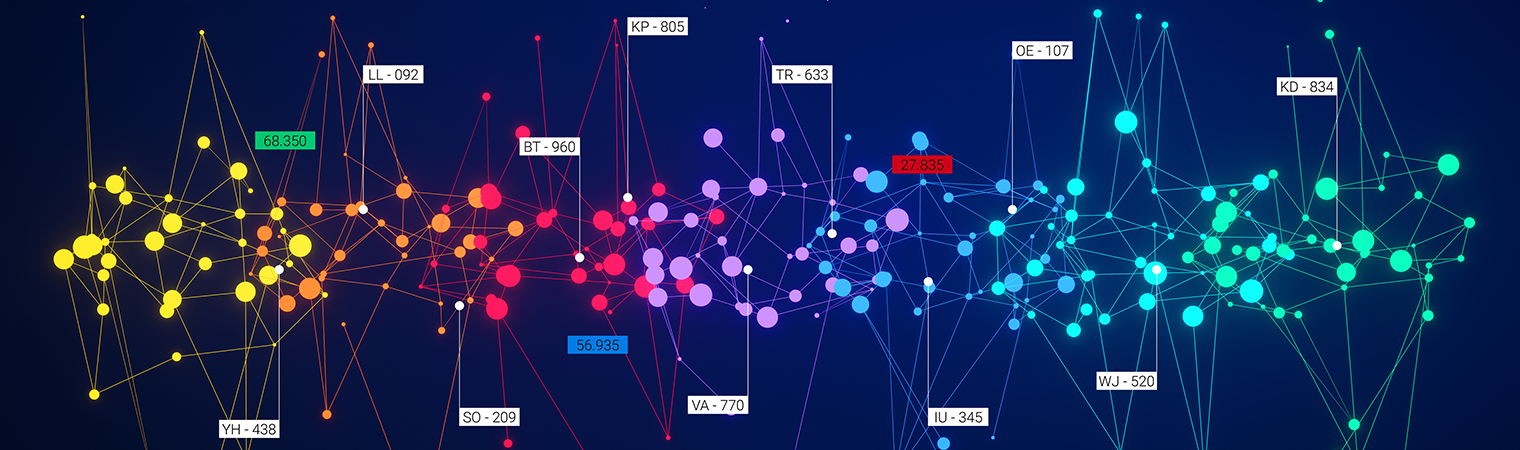
Private equity (PE) firms have been utilizing data analytics to optimize investment returns and drive value creation. This powerful tool can significantly enhance operational improvements, introduce new performance measurement and management tools, and have a meaningful impact on exit strategies. This article explores how data analytics can be leveraged to supplement the PE business model, focusing on operational improvements, performance measurement, and the potential impact on exit outcomes optimization. Together, these elements create a structured approach that aligns short-term actions with long-term goals, laying a foundation for sustained value creation and successful exits.
Finding Operational Improvements Through Data Collection and Benchmarking
Investors often give management sufficient operational freedom to run their businesses. Data analytics can provide investors with information needed to help improve operations while still giving management sufficient freedom to run their business. In this structure, where one party has a hand on the steering wheel and the other primarily relies on reporting, data tools can make this process more efficient, better informed, and collaborative.
In optimizing operations, one key area of impact is working capital optimization, which has been shown to deliver the most savings in the shortest timeframe. As simple as it sounds, analyzing transaction patterns, reaching out to vendors and customers to renegotiate payment terms, accelerating collections, or deferring payments does not require new infrastructure, lengthy implementation, or substantial investments. With access to data that is already collected or requires minimal incremental effort to collect, sales, margins, and product mixes can be improved with an implementation focus on high-margin opportunities.
Beyond financial metrics, business process optimization can be achieved through benchmarking data, such as staff productivity, process costs, and efficiency, which allows businesses to compare performance against industry peers and best practices. Functional benchmarking enables businesses to assess specific operational areas—such as supply chain management, procurement, or customer service—to help them pinpoint areas for improvement. While benchmarking will not give you a precise answer, it can highlight areas where additional attention may be needed, reducing months of work looking for efficiencies that may not exist.
The initial investment in data analytics is often relatively minimal. Modern ERP (Enterprise Resource Planning) systems are already capturing the data, so the information is available for analysis once the data points have been determined. With every project being unique, data analytics can sometimes deliver results in minutes, while other times it may take longer.
Case Study: Client Gets Back $850,000 in Less Than an Hour
Can you make $850,000 in less than one hour? Yes, with data analytics. This is a real story of a private equity-owned food manufacturing operation in Wisconsin who had just completed the acquisition of a competitor and was integrating the operations of the two businesses.
We supported the client with synergy assessment and data analytics. Within the first hour on-site, analyzing vendor transactions and payments, we identified one vendor whose payment terms were out of policy – they were paid upon receipt, the same day, for over a decade, while payment terms for others were generally congruent with the company policy—60 days. AP (Accounts Payable) days appeared good on average, but only a deeper dive into transactional data helped reveal this irregularity in a newly acquired business.
The working capital impact was significant—$850,000, identified within the first hour of working on data. An adjustment (which the vendor was amenable to) delivered immediate cash relief and helped the PE firm reduce leverage shortly after the acquisition.
Performance Measurement Systems for Informed Decision Making
Many PE firms rely on data analytics to monitor and improve performance of their portfolio companies. The capabilities of the performance measurement systems go well beyond financial metrics and can now include real time operational reporting, customer data and a sentiment analysis of customer feedback. Through detailed analysis of these metrics, PE firms can identify trends and make informed, data-driven decisions to improve financial health of their portfolio companies.
Another aspect of performance measurement, which is often overlooked, is benchmarking. In data analytics context, benchmarking extends beyond traditional financial metrics and enters operations territory with functional process cost, headcount, efficiency, and productivity data.
How many FTEs (full-time equivalents) should an AR (Accounts Receivable) function have for an Industrial products manufacturing industry? What is an average delinquency rate? What headcount should the IT (Information Technology) helpdesk have for 500 users? What is the comparable IT cost for a business of a given size in each industry?
As some practitioners say, this is not information, this is intelligence. This deeper, data-driven approach to benchmarking helps identify areas where the company is underperforming and provide insights into best practices that can be scaled up across the whole investment portfolio. At this point, you are developing an intangible asset–organizational know-how, which will keep working and saving daily. Contrary to the common belief, this solution is relatively low-cost and easy to deploy.
The AI capabilities of a data analytics toolset are on the other end of the complexity scale. While predictive analytics may be too complex in some instances and may yield to conventional spreadsheet tools in terms of efficiency, accuracy, and simplicity, Natural Language Processing (NLP) features can show the full power of data analytics. Tens or even hundreds of thousands of customer feedback forms can be analyzed and summarized, providing not only sentiment analysis (positive, negative, neutral) but also highlighting key insights that customers find most important.
These insights can include hidden quality issues, customer service bottlenecks, or new flavors or product features that customers are expecting. In today’s business world, this level of insight—listening to and hearing your customers, addressing their concerns, and meeting their needs—is a prerequisite for a successful investment story.
How Data Analytics Create a more Profitable and Efficient Exit Process
There is a logical link between operational improvements and exit valuations. Strong historical performance often translates into higher profitability (i.e., valuation base), while outlook and growth narrative drives the valuation multiple up. If done correctly, this creates a compounding effect, resulting in a competitive sale process which tends to generate higher returns. This sort of improvement is not a quick fix but is the culmination of concerted effort prior to a sale.
Investing in a data analytic-enabled performance improvement plan can not only make the operating period more profitable, it can also this exit process more efficient. With data-driven insights, PE firms can identify and implement efficiency gains, cost reductions, and revenue growth opportunities within their portfolio companies, allowing their teams to focus on what really matters.
Focused, operational enhancements combined with a performance measurement toolset not only improve the company’s financial performance but also make it more attractive to potential buyers, who are willing to pay a premium for a well-optimized and profitable business.
Five Hurdles Private Equity Firms Face when Adopting Data Analytics
Despite the advantages, PE firms face several challenges in leveraging data analytics effectively. Our team has identified five key challenges and included recommendations on how to mitigate them.
Common Challenges to Successful Implementation
- Availability of data: Portfolio companies often have disparate data systems, making it difficult to consolidate and analyze information. Implementing a centralized data repository can address this issue, ensuring that all relevant data is accessible and integrated. Automation tools can further streamline data collection and integration processes, reducing the time and effort required.
- Timeliness of reporting: Traditional reporting methods can be slow and prone to errors, leading to outdated or inaccurate data. Real-time data analytics platforms can solve this problem by providing up-to-date information and enabling faster decision-making. These platforms can automate data collection and reporting, ensuring that PE firms have access to accurate and timely data.
- Data accuracy: Inaccurate data can lead to flawed insights and poor investment outcomes. Implementing robust data validation and quality assurance processes can help ensure the accuracy and reliability of data. Partnering with trusted data providers can also enhance data quality and mitigate risks associated with inaccurate information.
- Benchmarking: Finding appropriate benchmarks can be challenging due to the unique characteristics of each investment. Using a combination of industry standards and custom benchmarks tailored to the specific context of each portfolio company can provide more meaningful comparisons. Data analytics can facilitate this process by identifying relevant benchmarks and providing insights into performance relative to these standards.
- Motivating the operating company: Data analytics can help by providing transparency and clear performance metrics. By tracking and sharing performance data, PE firms can create a culture of accountability and continuous improvement. Incentive structures tied to performance metrics can further align interests and motivate the team to achieve common goals.
Case Study: Finding Solutions for an Equipment Reseller
Here is a real-world example from a Capstone equipment reseller client that had a record-breaking year in sales but struggled with cash flow and storage space. Despite strong revenue, too much capital was tied up in slow-moving inventory, and the supply chain wasn’t as efficient as it could be. The company needed to free up cash, make better purchasing decisions, and use its warehouse more efficiently.
A closer look at the company’s inventory data showed several issues. Many products weren’t selling, yet they took up valuable warehouse space. Purchasing decisions weren’t aligned with demand, leading to an oversupply of machines that took too long to sell. On top of that, unreliable data made it hard to forecast sales and manage stock properly.
Capstone completed an analysis of sales and inventory records, which helped triangulate problem areas and find opportunities for improvement. Cleaning up the data helped spot which products weren’t moving and which ones customers actually wanted. We identified and recommended reducing stock on items that had never sold before and adjusting purchases to focus on products with a quicker turnaround. These changes made it easier to manage inventory and improve transactional velocity. At the same time, better warehouse organization reduced storage costs and increased efficiency.
These adjustments had a real impact. Sales moved faster, adding more than $700,000 to annual profit. Cutting down on stagnant inventory freed up $1.2 million in cash, giving the company more working capital flexibility. The warehouse also became more efficient, with a 17% reduction in space usage, lowering costs further.
Conclusion
Data analytics is one of the most powerful tools available to enhance the private equity business model by providing deeper insights, improving operational efficiency, and optimizing exits. Private equity firms that incorporate data-driven insights into decision-making can create more value, improve performance, and achieve higher returns for their investors. The integration of data analytics into the investment horizon and operational control ensures that performance metrics align short-term actions with long-term goals, leading to sustained value creation and successful exits.
PE firms face several challenges in leveraging data analytics effectively, such as data availability, timeliness of reporting, accuracy, benchmarking, and aligning interests with operating company teams. However, a combination of centralized data repositories, real-time analytics platforms, robust data validation processes, and transparent performance metrics can address these challenges. Expanding their data capabilities allows PE firms to unlock significant value and drive superior performance across their portfolio companies.
If you have questions about any of these concepts and how they would apply to your specific circumstances, please contact a member of our Financial Advisory Services (FAS) group’s Performance Improvement team.
Insights for Middle Market Leaders
Receive email updates with our proprietary data, reports, and insights as they’re published for the industries that matter to you most.